Series:
Essay #3:
Synopsis:
Causation
Probability
Probabilistic causation is a useful abstraction for predicting the behavior of simple systems
In my last essay, I showed the classic, mechanical view of causation. The classic view is of matter in motion, going forward in chains of cause-and-effect like billiard balls on a pool table. Then we encountered randomness and complexity which messed everything up.
Probabilities are the next level of abstraction after mechanical causation. Erwin Schrodinger, in his 1943 book What is Life? makes an example of fog in a glass container. Fog is made of water droplets. Schrodinger wanted to explain the macro-behavior of fog based on the micro-behavior of its water droplets; he wanted one causal law that worked across the two levels of complexity. The problem was that when Schrodinger watched an individual droplet, he saw it move randomly due to Brownian movement. He had no idea what the droplet would do next, which meant he couldn’t make causal predictions from the behavior of the droplet. It was like predicting the perambulations of a flea on a dog.
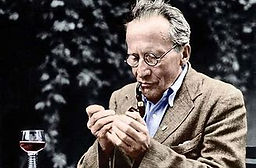
In brief, to derive causal laws for the behavior of fog (the aggregate), Schrodinger began by studying a water droplet (the individual), but the individual moved randomly and broke his causal chain. So Schrodinger changed his perspective to the fog, not the droplet, and found that fog moved by easily defined laws: fog sinks lower in the container at a defined velocity based on such factors as gravity and viscosity of air.
As a general proposition, the more individual and granular my vision, the greater the effects of randomness, and the lesser my ability to formulate laws to describe behavior. I get physical laws when I move to an aggregate level of organization, said laws being a statistical probability of the behavior of the aggregate. In a roomful of air molecules, I won’t know where any individual molecule is, but if the data set is big enough, I can safely predict that the aggregate will spread out evenly, enabling me to breathe in all corners of the room, although there exists a small chance that all the molecules will evacuate one corner leaving me to suffocate there.
​
Nassim Taleb gives the best example. Over time, random events regress to the mean, leaving a person’s substantive traits to shape the general outcome of his life. When it’s all over, the lucky fool who won the lottery once then kept on playing, likely will die in about the same place as if he’d never won the lottery that one time. Contrast the dentist who drills teeth 5 days a week for 30 years, keeps a modest budget, and puts his money in safe investments. The dentist likely will die with a lot more money than the lottery-winning fool.
A probability is a prediction based on limited data. I'm betting that randomness over here cancels the randomness over there, and the next one will be like the last one. Remember, when I have perfect data, hooked into God’s mind, I use a classical, mechanistic theory to derive an answer of absolute certainty. With less than perfect data, I make inferences based on the data I have.
Everyone thinks in probabilities. Usually it’s a quick and dirty heuristic to predict the possibility of outcomes and take action. When I describe my car’s overheating to my mechanic, Mr.E, he’s already ranked the causes by probability before I finish the sentence. He fixes the car by going through the probabilities in order of priority until he finds the culprit; probably the radiator. When Mr.E finishes the job, he shows me what went wrong, tracing causation from beginning to end in classical, mechanistic fashion. That is, before he’s looked at the car, Mr.E analyzes the problem by probability, but after he’s fixed the car, he explains it by mechanical determinism. I guess for Mr.E, the future is a probability while the past is determined.

A probabilistic theory is an abstraction, one step removed from real, individual things. That’s a strength because a good abstraction speaks to the general characteristics of a population. When thinking about my car, Mr.E initially thinks of how car radiators generally behave. From this perspective, an abstraction is realer than reality like a great novel is realer than real life. It’s also a weakness, because probabilities say little of the individual, a fact of which, as an ethical principle, I try to be mindful. Schrodinger gives the example of a chemist working with a large number of the same type of molecule in vitro. The chemist can tell you that after the elapse of X minutes, Y% of the molecules likely will have reacted, but he can’t predict which molecule will react and which won’t. The individual is real-in-itself and mostly useless in analytical thought, whereas the probability is an abstraction of manifold utility.
We’ve fallen in love with our probabilities, and rightly so, because the inanimate world rewards our love with accurate predictions. For fog, a probability is easy to calculate today and it’ll be just as accurate in 1,000 years. But don’t expect the same treatment from living organisms and complex systems, which are subjects I address at length in later essays. Probabilities don’t work so well in complexity, mainly because there’s no simple and segregated aggregate from which to generate a statistical probability. The problem is that, in the mess of complexity, we never know what the statistic really applies to, what it points to. Probabilistic causal theories can’t catch up with complexity. For my part, I side with Winston Churchill: “the only statistics you can trust are the ones you falsified yourself.”
The laws for the individual and for the aggregate are different. When looking from the individual to the aggregate, we move across different levels of complexity where each level requires a different causal theory. It’s the same old moral: reach into the toolbox of causal theories for the right theory at the right level of complexity. It’s also a rule of relevancy. To understand the individual, like a water droplet, look at the individual (most likely with a mechanistic theory). To understand a simple aggregate, like fog, look at the aggregate (using a probabilistic theory). To understand fog as it exists in a complex system, like the Earth’s atmosphere, look at the entire, complex whole… and prepare to let go of your mechanistic and probabilistic theories.
Martin Buber said, “Causality has an unlimited reign in the world of It.” Remember my image of reality as material parts that bounce around alone then assemble like Lego blocks into more complex things? That’s the image behind mechanistic and probabilistic causation: brute matter operated on by physical forces and laws. This is the It-perspective of science, and it’s good for analyzing simple systems… but not so good for complex systems. Complexity is their kryptonite.
Essays in this Series, Causation:
​